A focus on measures & evidence-based science is important
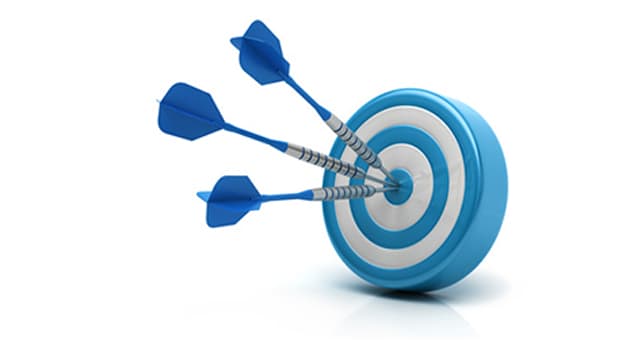
According to a CEB survey, only 18 percent of business leaders say they trust their talent data. A staggering 82 percent don’t believe their talent analytics focus on the right issues, and only 15 percent of HR leaders say they have made a business decision based on insights gleaned from HR analytics. Yet, investment in talent analytics globally is upwards of 600 million dollars. How can organizations derive greater value from their people analytics practice? In this article, I present the thesis that organizations that avoid some of the common pitfalls, adopt an evidence-based focus, and deliver personalized insights from analytics will be better positioned to fulfill the promise that talent analytics has to offer. If done right, it can be of significant competitive advantage to organizations. Imagine a learning plan that is automatically created based on the future skill needs and aspirations of an employee. How about the intelligent identification of rotational opportunities to grow your future leaders? Imagine automatic rescheduling of tasks for optimal time utilization, and intelligent calendar management to promote work-life balance. The opportunities are tremendous, and the journey for most organizations has just begun.
Talent analytics is a mechanism to uncover insights from people and business data that might otherwise be invisible through pure observations. To be of utility, these insights must align with the critical priorities of the organization. Better still, they must not just describe the current state but provide a forward-looking view of what’s to come. This is the value of predictive and prescriptive analytics. A review of HR practices shows that the vast majority of the people operations such as hiring, development, and performance management have largely been intuition-led. This is likely the reason why HR has struggled to earn the credibility of our counterparts in manufacturing, finance, or marketing, who have a longer history of data-based decision making. HR has an equal or better opportunity to be analytics-led. Ultimately, all HR activities generate data about people – attendance, absence, time spent to more abstract concepts like trust, leadership, and influence. In fact, we have 150 years of quantified science supplying a deep understanding of human behavior in the workplace. However, there has been limited adoption of this knowledge and understanding. Below I elaborate on some of the most limiting challenges that organizations must overcome.
- Many organizations rely on a centralized analytics function. Given the scarcity in good data science expertise, this may seem like a prudent choice. However, such an organizational design comes with its limitation. To conduct timely and meaningful analytics, it is important to spot and capture opportunities, such that they can be viewed from an analytical perspective. It is also beneficial to combine analytics with experimentation. Organizations that have a centralized analytics function tend to engage these teams when there is data available to be analyzed. This often is too late. It offers fewer opportunities to influence the program design and data collection plan. As a result, the analytics are more descriptive than predictive.
- The second challenge relates to over-reliance on past company data. Organizations spend countless hours mining their employee engagement surveys to understand engagement drivers. In one such exercise, data scientists found that of the five practices that were evaluated in their survey, employees who had the option to apply for jobs internally were more engaged. Based on this finding, the company decided that managers must encourage employees to look for positions outside their immediate function. One challenge with this approach could be a false cause-effect attribution. It also narrows intervention options to only those practices that the company may have tried in the past or considered in the survey. A better approach would be to look at the predictors of engagement, and select interventions that have a higher probability of delivering the desired outcome. In the case of engagement, autonomy has repeatedly been found to be among the more efficacious predictors. Basing interventions on prior evidence and designing experiments to find the best alternative may be a better use of the analytics functions. The practice of conducting AB testing in marketing leverages a similar idea of experimentation.
- The third challenge pertains to the assumption that to perform impactful analytics one requires big data. This is a fallacy and one plaguing not just HR but the broader business world. With increasing digitization, data velocity and variety is no longer a challenge. A learning management system can generate 1000 data points per user with just 10 minutes of interactions. Even when big data is available, the challenge is in finding the signal in the noise. That is, making sense of the data in a way that each stakeholder can take meaningful action. Additionally, to do good talent analytics, organizations need more than just data (information residing in various systems which can be counted). Organizations must invest in capturing abstract constructs in a quantitative format called measures. A measure provides ruler-like properties to abstract phenomenon (e.g. risk, trust, leadership, making them amenable to sophisticated analyses. For example, in a learning platform, it is easy to capture transactional data pertaining to time spent and activities attempted, but these may not predict learning and skill development. To measure skill one must measure concepts such as knowledge structures and self-efficacy.
To overcome some of these challenges, it is important to evaluate the organizational structure and tighten the integration between the talent analytics team and the HR function.
Secondly, the organization must invest in developing specialized talent analytics skills that go beyond statistics, data science, and software programming.
Talent analytics team members must have a sound understanding of the science of human performance and evidence-based methods. They must also have expertise in specialized statistical techniques relevant for measuring and analyzing people data such as scaling and hierarchical linear modeling.
As we look to the future, I see talent analytics efforts pivot in three important ways, (a) maturing from administration support tool to performance augmentation system, (b) evolving from describing the past to providing future-focused insights, (c) transitioning from intuitions-led biased decision makers to creating meritocratic workplaces managed through facts.
Historically, talent analytics has been used to support administrative decisions, for instance demonstrating program impact and summarizing workforce characteristics (e.g. gender distribution), all primarily designed to support administrative decision making. In the future, we will see greater use of analytics to augment employee performance. The language editing tool, Grammarly, is one such example. It reviews writing habits and provides real-time grammar suggestions and learning tips. Imagine similar tools that help a manager prepare for a motivating performance review meeting, or prepare to negotiate with a difficult client. The potential for such methods in unlocking productivity is remarkable. To provide personalized performance support, analytics must not just focus on the past data but reliably predict what one is likely to do. This is the benefit of prescriptive analytics that can find the best option across multiple future states.
Prediction and prescription are some of the key reasons why focus on measures, and evidence-based science is important.
The net result of using analytics that provides performance support and presents a forward-looking view is in creating an equal playing ground where the best can rise to the top. I am personally most excited about the potential of talent analytics in creating fair and equitable workplaces, where meritocracy can thrive.
In conclusion, I see talent analytics as the third eye and the sixth sense. It can help you see what’s not readily visible, enable better sensing, and power your abilities with greater intelligence. I leave you with this question: If the future state I describe were to come true, how would your life be different? If we can imagine a better future, we can create it.