Outlook 2025: The most promising AI trends today
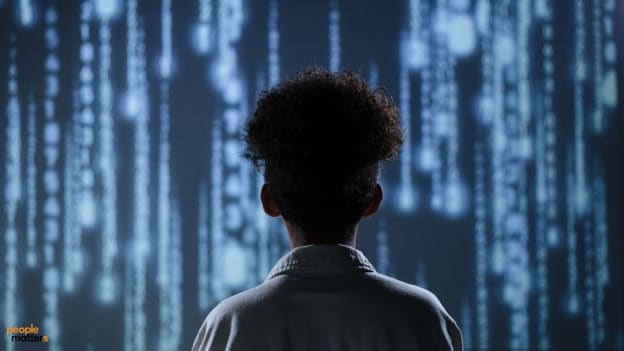
Much like the early days of computing, the generative AI landscape is shifting from being an exclusive tool for large organisations to something that can be used by almost anyone, regardless of technical background.
Last year, People Matters Global predicted that, in 2024, AI would hit the fast lane. And indeed, experts have been hard at work to determine how this leap in technology could be integrated into our daily lives.
This will only accelerate in 2025.
The use of AI has become even more ubiquitous this year. According to PwC, about 73% of companies in the US, for example, apply AI in their organisation.
Meanwhile, a separate study by Deloitte revealed that students and employees in Southeast Asia use generative AI widely, with high growth expected in the years to come.
One study, however, found that only 23% of organisations in Southeast Asia take on a "transformative" approach to their AI adoption, meaning they have long-term plans and use AI to create new business models and products. This suggests that while many companies may be exploring AI, a smaller percentage are fully integrating it into their core strategies.
The initial excitement around generative AI is giving way to a more practical and measured approach. Instead of just experimenting, businesses are now focused on using generative AI to solve real-world problems.
This shift is leading to more thoughtful and cautious strategies for developing and deploying AI, with a strong emphasis on ethical considerations, safety, and upcoming regulations.
The rapid evolution of Generative AI
Generative AI is evolving at an unprecedented pace, mirroring the trajectory of computer technology but accelerating far more quickly.
Much like the early days of computing, the generative AI landscape is shifting from being an exclusive tool for large organisations to something that can be used by almost anyone, regardless of technical background. This process of democratisation is unfolding in distinct stages that echo the rise of personal computing.
Also Read: AI-driven teamwork – 4 trends in 2024
We’re now entering what can be described as a “hobbyist” phase in the development of generative AI. Individuals are beginning to experiment with AI tools in new and creative ways.
Open-source platforms, user-friendly interfaces, and cloud-based computing have made it easier for anyone with an interest in the field to engage with generative AI.
Looking further ahead, the future of generative AI appears to be one of widespread democratisation. In the coming years, we can expect generative AI to be a tool available to everyone, empowering individuals and organisations of all sizes and technical abilities.
Also Read: How AI in HR hit the fast lane to digital transformation
The most promising trends in AI today
1) Multimodal AI
Instead of just processing one type of data like text, imagine AI that understands images and sounds too. This "multimodal" approach is a big step towards AI that can experience the world more like humans do, by processing diverse sensory information.
As Mark Chen from OpenAI put it, "The interfaces of the world are multimodal. We want our models to see what we see and hear what we hear." This means AI that can not only understand our world but also create content that engages multiple senses.
Imagine snapping a photo of your fridge and having AI instantly suggest recipes based on what's inside. That's the power of multimodal AI.
OpenAI's GPT-4 can process both images and audio, allowing for more natural and intuitive interactions. Instead of just typing a request, you could even ask GPT-4 aloud using its voice mode, making it feel more like a conversation with a helpful assistant.
While most generative AI efforts today focus on text, Matt Barrington, Americas emerging technologies leader at EY, believes the true potential will be realised when text, conversation, images, and video are combined. "The real power comes from cross-pollinating all three and applying them across various industries," he said.
Multimodal AI is finding a wide range of real-world applications, with its impact continuing to grow. In healthcare, for instance, these models can analyse medical images alongside patient history and genetic data to enhance diagnostic accuracy.
On a broader scale, multimodal models are also expanding the capabilities of employees across different job functions, enabling individuals without formal design or coding expertise to engage in tasks traditionally reserved for specialists.
Introducing multimodal capabilities could enhance models by providing new sources of data for learning. According to Chen, as multimodal models improve at processing language and begin to reach the limits of what they can learn from text alone, they aim to give them access to raw data from the world, such as video and audio, allowing them to perceive and make inferences independently.
2) Agentic AI
Agentic AI represents a major shift from reactive to proactive systems. These advanced AI agents possess autonomy, proactivity, and the ability to act independently.
Unlike traditional AI systems, which primarily respond to user inputs and follow pre-programmed instructions, AI agents are designed to understand their environment, set goals, and take actions to achieve those objectives without requiring constant human intervention.
For instance, in environmental monitoring, an AI agent could be trained to gather data, identify patterns, and initiate preventative actions, such as detecting early signs of a forest fire.
Similarly, a financial AI agent could actively manage an investment portfolio, using adaptive strategies to respond to real-time changes in the market.
As computer scientist Peter Norvig, a fellow at Stanford’s Human-Centred AI Institute, noted in a recent blog post, “2023 was the year of chatting with AI. In 2024, we’ll see agents that can get things done for you—like making reservations, planning trips, or connecting to other services.”
Moreover, the combination of agentic and multimodal AI could unlock new possibilities. Chen, in a recent presentation, illustrated how a multimodal, agentic AI could simplify tasks like building an image recognition app. Previously, creating such an app required training a custom model and figuring out deployment.
Now, with multimodal agentic models, this process can be achieved through simple natural language prompts.
3) Open source AI
Building powerful AI systems, particularly large language models (LLMs), is a highly resource-intensive and expensive endeavour. For a long time, only tech giants with vast computing power and financial resources could afford to develop such advanced models.
However, the rise of open source AI is changing this landscape, offering a new way for anyone—from small startups to independent researchers—to access and contribute to the development of cutting-edge AI technologies.
Open source AI can be thought of as a shared toolbox for building artificial intelligence. Unlike proprietary systems that are locked behind paywalls, open source models are freely available to anyone who wants to use them. This means that individuals and organisations, regardless of their size or budget, can experiment with, modify, and build upon the work of others. By lowering the cost and technical barriers to entry, open source AI is making it significantly easier for a broader range of people to engage with this powerful technology.
This democratisation of AI access has several important implications. First, it enables more people to enter the field.
Amaller companies and independent researchers who previously lacked the resources to develop their own AI models can now leverage existing open source tools. This creates an environment where innovation is no longer limited to a few major players but is accessible to a much wider community.
For many, this marks a crucial opportunity to create new products, conduct research, or even start new businesses using AI without the prohibitive costs associated with proprietary systems.
Second, open source AI accelerates innovation. Because the AI community can freely build on one another's work, the pace of advancement is much faster.
Researchers can take existing models, improve them, and quickly share their results with others, leading to rapid iterative progress. Collaboration across different organisations and individuals also fosters the development of better and more diverse solutions, benefiting the entire ecosystem.
Finally, open source AI promotes transparency and accountability. By making models and their underlying code publicly available, it becomes easier to spot potential ethical issues, such as biases in the data or flaws in security. This transparency encourages responsible development and helps ensure that AI technologies are built in a way that prioritises fairness, safety, and security.
Despite concerns about potential misuse, the rise of open source AI is undoubtedly a transformative force, democratising access to this powerful technology and fostering a new wave of innovation.
Through greater accessibility, faster innovation, and enhanced transparency, open source AI is opening up new possibilities for everyone.
4) Retrieval augmented generation
While generative AI tools saw widespread adoption in 2023, they are still hindered by the issue of hallucinations—plausible but inaccurate responses to user queries. This limitation has been a significant barrier to enterprise adoption, especially in business-critical or customer-facing scenarios where errors could be catastrophic.
Retrieval-augmented generation (RAG) has emerged as a promising technique to mitigate hallucinations, offering potentially transformative benefits for enterprise AI deployment.
RAG combines text generation with information retrieval to improve the accuracy and relevance of AI-generated content. By allowing large language models (LLMs) to access external information, it helps produce more precise and contextually aware responses.
This approach also eliminates the need to store all knowledge directly within the model, which reduces its size, increases processing speed, and lowers costs.
As Barrington explained, "RAG allows you to gather vast amounts of unstructured information—documents, etc.—and feed it into a model without the need for fine-tuning or custom training."
These advantages are especially valuable for enterprise applications that rely on up-to-date, accurate information. For instance, businesses can leverage RAG alongside foundational models to develop more efficient and informative chatbots or virtual assistants.
5) Customised enterprise generative AI models
While general-purpose tools like Midjourney and ChatGPT have gained widespread attention among consumers, businesses may find that smaller, niche AI models offer more lasting value.
As demand grows for AI systems tailored to specific needs, customised generative AI models—modified from existing systems—are likely to become the preferred solution for many organisations.
Building a new model from scratch is often resource-intensive, so most companies opt to fine-tune existing models on domain-specific datasets to make them more cost-effective and manageable.
Shane Luke, VP of AI and machine learning at Workday, points out the high costs and latency involved in using large public models like GPT-4 via API. "Calls to GPT-4 are very expensive, both in terms of cost and latency," he said.
"We're working on optimising so that we have the same capability, but it's very targeted and specific, with smaller models that are more manageable."
The main advantage of customised generative AI is its ability to address niche business requirements. Tailored models can be developed for specific use cases, such as customer support, supply chain management, or document review – especially in sectors with specialised terminology like healthcare, finance, and legal services.
Also Read: Are Singaporeans reluctant to upskill in AI?
Regulation and ethics of AI
As AI continues to proliferate globally, the importance of managing associated risks becomes increasingly critical. Government bodies and organisations like OpenAI are working to ensure that AI is developed and deployed responsibly and ethically.
In March 2024, the European Union advanced a landmark AI regulation aimed at addressing consumer concerns and setting a legal framework for AI use. Expected to become law later this year, the bill underscores the need for comprehensive oversight.
Without proper regulation, AI systems could exacerbate issues like data manipulation, misinformation, bias, and privacy violations, posing significant societal risks. For instance, AI tools could perpetuate discrimination or legal challenges if they fail to collect data that accurately reflects diverse populations.
Furthermore, the global reach of generators like ChatGPT has led to copyright disputes, with companies and publications suing OpenAI for infringement. Ensuring AI operates within clear ethical boundaries and legal frameworks is essential to mitigating these risks and fostering trust in the technology.