Why the AI reality is falling short of expectations
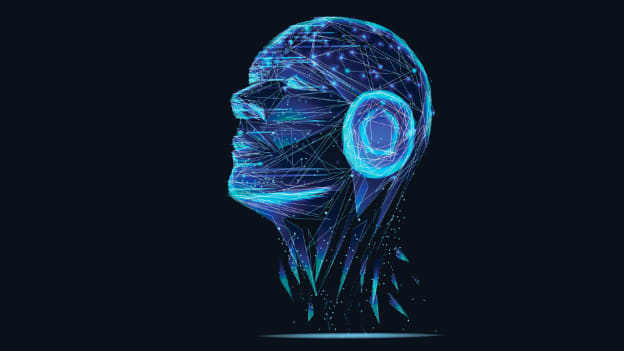
With the Coronavirus taking over the world, one thing that has gone silent is the various experiments performed in the field of AI and how it is a potential threat to humans posed by AI. It is amusing to see that one of the most sought-after technology isn’t playing the major role some may have hoped for.
“This (pandemic) is showing what bulls--t most AI hype is. It’s great and it will be useful one day but it’s not surprising in a pandemic that we fall back on tried and tested techniques,” said Neil Lawrence, the former director of machine learning at Amazon Cambridge.1
Around the world, AI which was one of the most coveted and talked about technologies is recently, increasingly being questioned about its usefulness and ability to drive business outcomes.
When it comes to making the business run better, AI has shown more promises than performance. According to International Data Corporation’s survey, global organizations that are already using AI solutions found only 25 percent have developed an enterprise-wide AI strategy. Most organizations reported failures among their AI projects, with a quarter of them reporting up to a 50 percent failure rate.2
Why? Too many times, AI fails to deliver the positive impact that businesses really want from the technology, like more revenue, lower cost, fewer customers lost to churn, higher manufacturing quality, and lower waste and fraud.
Rather, the outcomes that we are receiving are inaccuracies.
Inaccuracies, a lot of inaccuracies
In May 2020, Microsoft laid-off dozens of journalists and editorial workers at its Microsoft News and MSN organizations. The layoffs are part of a bigger push by Microsoft to rely on artificial intelligence to pick news and content that’s presented on MSN.com, inside Microsoft’s Edge browser, and in the company’s various Microsoft News apps. Many of the affected workers are part of Microsoft’s SANE (search, ads, News, Edge) division, and are contracted as human editors to help pick stories.
However, according to recent developments, Microsoft’s AI editors are already showing signs of inaccuracy.
The AI reportedly used a photo of Leigh-Anne Pinnock on a story about her fellow bandmate Jade Thirlwall’s experience. Thirlwall criticized the company through her Twitter, tagging MSN, Microsoft’s news publishing website on her post. “@MSN If you’re going to copy and paste articles from other accurate media outlets, you might want to make sure you’re using an image of the correct mixed-race member of the group," she wrote.
Needless to say, it was one of the major failures on Microsoft’s part to replace humans with AI.
A machine with inherent bias?
AI and ML have a huge bias problem and while most companies wouldn’t have noticed, Amazon ditched its AI-based recruitment technology as what they discovered was the technology had an inherent bias against black people and women.
Or rather, they have a huge problem with bias. And the launch, drama, and subsequent ditching of Amazon’s AI for recruitment is the perfect poster-child.
Amazon planned to go big with their recruitment technology where they claimed in media that they literally wanted it to be an engine where they going to give AI 100 résumés and it will spit out the top five, and the company will hire those.
But eventually, the Amazon engineers realized that they’d taught their own AI that male candidates were automatically better. Amazon trained their AI on engineering job applicant résumés. And then they benchmarked that training data set against current engineering employees.
So, from its training data, Amazon’s AI for recruitment realized that candidates who seemed whiter and more male were more likely to be good fits for engineering jobs.
According to International Data Corporation's survey, global organizations that are already using AI solutions found only 25 percent have developed an enterprise-wide AI strategy
Automating conversation with no transformational output
Before we delve deeper into this topic, it is time to put yourself in reverse mode and remember the scene where you were raising a complaint about the online delivery of a product on the company’s chatbot. The whole process seems as if chatbots aren’t valuing people’s time. Most consumers feel as though chatbots talk in circles and give them the runaround until eventually telling them to call a customer service number – wasting their time and increasing their frustration with the brand.
Everyone was ready for the era of the chatbot. Then it all fizzled out. We’d fallen fool to another AI hype cycle.
One of the main causes of reality falling short of the chatbot hype was the lack of AI ability available to the bots. That is, they were a lot less ‘smart’ than people thought.
Instead of intelligent conversation, customers met a brick wall in the form of confused chatbot error messages.
The problem was that, due to this mis-assigned intelligence, chatbots gained too much responsibility too fast. All too often, businesses deployed their shiny new chatbot as a standalone service option. And, as a standalone contact channel, chatbots simply weren’t functional enough.
A standalone chatbot isn’t capable of anything an FAQ section with a search function can’t do. They can provide a conversational interface for customers with frequent questions. But it relies on the customer asking the right questions. They can’t take on the challenges that a human agent can. In short, chatbots used as a standalone didn’t add enough value to warrant the disruption they caused. When used instead of a human agent, they fell short. And so too did the chatbot hype.
Can businesses rely on AI for making decisions?
Conventional AI solutions operate inside “black boxes,” unable to explain or substantiate their reasoning or decisions. These solutions depend on intricate neural networks that are too complex for people to understand. Companies utilizing conventional AI approaches primarily are in somewhat of a quandary because they don’t know how or why the system produces its conclusions, and most AI firms refuse to divulge, or are unable to divulge, the inner workings of their technology.
However, these “smart” systems aren’t generally all that smart. They can process very large, complex data sets, but cannot employ human-like reasoning or problem-solving. They “see” data as a series of numbers, label those numbers based on how they were trained, and depend on recognition to solve problems. When presented with data, a conventional AI system asks itself if it has seen the information before and, if so, how it labeled that data last time. It cannot diagnose or solve problems in real-time unless it has the ability to communicate with human operators.
Scenarios do exist where AI users may not be as concerned about collecting information around reasoning because the consequences of a negative outcome are minimal, such as algorithms that recommend items based on consumers’ purchasing or viewing history. However, trusting the decisions of black box-oriented AI is extremely problematic in high-value, high-risk industries such as finance, healthcare, and energy where machines may be tasked to make recommendations on which millions of dollars, or the safety and well being of humans, hang in the balance. The AI community is thinking long and hard about how it can make itself more useful. Just relying solely on AI will lead us nowhere.
According to the World Economic Forum, efforts to leverage AI tools in the time of COVID-19 will be most effective when they involve the input and collaboration of humans in several different roles.4
The data scientists who code AI systems play an important role because they know what AI can do and, just as importantly, what it can’t. We also need domain experts who understand the nature of the problem and can identify where past training data might still be relevant today. Finally, we need out-of-the-box thinkers who push us to move beyond our assumptions and can see surprising connections.
References:
[1] Procaccia, A. Bloomberg. (2019). Are you a robot?
[2] IDC: The premier global market intelligence company. (n.d.).(2019). IDC Survey Finds Artificial Intelligence to be a Priority for Organizations But Few Have Implemented an Enterprise-Wide Strategy.
[3] Sharma, A. and Ltd, P.M.M.P. (2020). Another failed attempt of AI replacing humans: Microsoft AI Editor already shows signs of inaccuracies.
[4] Blier, N. (2020). Stories of AI Failure and How to Avoid Similar AI Fails.
[5] Matissa Hollister (2020). COVID-19: AI can help - but the right human input is key.